What is Generative AI: Understanding the Next Wave of Artificial Intelligence
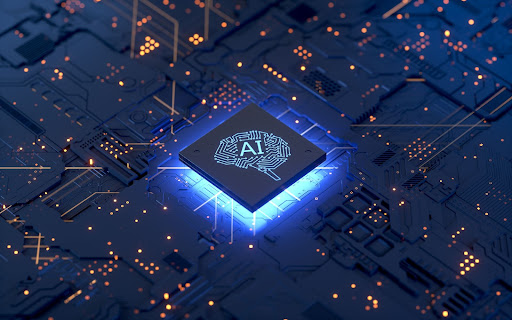
Artificial Intelligence (AI) has come a long way since its inception, from machine learning to deep learning and neural networks. But, have you heard about Generative AI? Generative AI is the future of AI, and it’s changing the way we interact with technology. In this post, we’ll take a closer look at generative AI and understand how it works its applications, and its impact on the future.
What is Generative AI?
Generative AI Definition: Generative AI is a type of artificial intelligence that is designed to create new and original content. It is a subset of machine learning and uses a technique called generative models, which allows AI algorithms to generate new data that is similar to the input data it has been trained on. Generative AI can create new content such as images, music, video, text, and even entire websites.
How does Generative AI Work?
As mentioned, generative AI works by using “generative models”, which are algorithms that learn patterns and features from a dataset (training data) and generate new data that is similar to the input data. The most commonly used generative models are GANs (Generative Adversarial Networks) and VAEs (Variational Autoencoders).
Generative AI Models Explained
Generative AI models, also known as generative models, are a class of artificial intelligence algorithms that are designed to generate new content that is similar to the data they were trained on. There are various types of generative AI models, but two commonly used approaches are:
GANs work by training two neural networks simultaneously, one generator and one discriminator. The generator creates new data, and the discriminator evaluates how realistic the generated data is. The two networks compete against each other, with the generator attempting to generate data that the discriminator will classify as real. This adversarial training process leads to the generation of increasingly realistic samples by the generator.
VAEs are generative models that are capable of learning latent representations of the training data. They consist of an encoder network that maps input data to a latent space, and a decoder network that reconstructs the input data from the latent space. VAEs work by learning the distribution of the input data and then generating new data that follows the same distribution. By learning probability distribution over the latent space, VAEs generate new samples by sampling from this distribution and decoding the samples into the original data domain. VAEs are commonly used in image and video generation.
Real-Life Applications of Generative AI
- Art and Design: GenAI can create new and unique art, music, and designs, and even assist designers in creating new designs.
- Gaming: GenAI can create new levels, characters, and scenarios in games, making them more engaging and interactive.
- Marketing and Advertising: GenAI can help in creating personalized marketing campaigns and advertisements, targeting specific demographics.
- Fashion and Retail: GenAI can create new clothing designs and even help in predicting fashion trends.
- Healthcare: GenAI can assist in medical research by creating new drug compounds and analyzing medical images.
- Technology: GenAI can help in writing documentation and even in coding projects.
- Customer Service: Generative AI can handle customer and internal queries when trained on knowledge bases.
ChatGPT Made Generative AI Mainstream
Generative AI was introduced in the 1960s in chatbots. ELIZA was one of the first chatbot programs ever created, developed in the 1960s by Joseph Weizenbaum at MIT. It was designed to mimic the conversational style of a Rogerian psychotherapist, using natural language processing techniques to generate responses based on patterns in the user’s input.
ELIZA worked by parsing the user’s input and looking for specific keywords or phrases, then using a set of pre-written rules to generate an appropriate response. For example, if a user said “I feel sad,” ELIZA might respond with “Why do you feel sad?” or “Tell me more about your feelings.”
ELIZA was not capable of understanding the meaning of the user’s input or engaging in true conversation, but it was a groundbreaking achievement in the field of natural language processing and helped inspire further research in the area of artificial intelligence.
But it was not until the introduction of generative adversarial networks in 2014 that Generative AI could create convincingly authentic images, videos, and audio. When ChatGPT, a chatbot that Microsoft-backed OpenAI, was released in late 2022 it showed how powerful modern Generative AI could be.
ChatGPT has had a significant impact on natural language processing and artificial intelligence as a whole. ChatGPT has helped to advance the field of natural language processing by providing a powerful tool for understanding and generating human-like language. Its ability to generate high-quality text has led to a significant understanding of the impact of Generative AI. It is already being used to assist with many language processing tasks, such as machine translation, sentiment analysis, and text summarization.
Large Language Models
The AI powering ChatGPT is known as a large language model because it takes in a text prompt and from that writes a human-like response. A large language model is a type of AI system that is designed to understand and generate human language. It is called “large” because it has been trained on massive amounts of data, often using deep learning techniques and neural networks.
A large language model takes in text input and generates an output that is likely to follow the input text in terms of grammar, syntax, and meaning. Large language models have become increasingly sophisticated over the years, as advancements in machine learning and natural language processing have made it possible to train models on larger and more diverse datasets.
Large language models have been used in a wide range of applications, such as chatbots, language translation, and content creation, and even as an aid to help people with disabilities communicate. They are considered a major breakthrough in natural language processing and have the potential to transform many areas of human-machine interaction.
The Generative AI Market Today
Since September 2021, the generative AI market has experienced significant growth and shown immense potential across various industries– and the market dynamics are changing rapidly.
The growth of the generative AI market was driven by several factors, including advancements in deep learning algorithms, increased computing power, and the availability of large datasets for training. Some key observations about the generative AI market today include:
- Industry Adoption: Generative AI models were being adopted by a wide range of industries. For example, as mentioned in the creative sector, generative AI was used for content creation, such as generating realistic images, designing products, and composing music.
- Startups and Research: Numerous startups focused on generative AI emerged, aiming to develop innovative applications and solutions. Academic and industrial research communities were also actively exploring and pushing the boundaries of genAI, leading to new techniques and advancements.
- Generative Design: The use of generative AI in design and architecture gained traction. It allowed designers to explore vast design spaces, generate optimized and novel design solutions, and improve the efficiency and sustainability of structures
- Adoption Challenges: While genAI presents tremendous potential, there are several challenges that need to be addressed for widespread adoption. These challenges include the interpretability and explainability of generative models, ensuring ethical use of the technology, addressing biases in training data, and developing methods to control and guide the output of generative models
- Future Opportunities: The genAI market is expected to continue expanding as the technology matures and more industries recognize its potential. Advancements in areas such as controllable generation, unsupervised learning, and reinforcement learning hold promise for further enhancing generative AI capabilities and opening up new application.
The field of AI is dynamic, and new developments and trends emerge regularly, shaping the market landscape. Market research reports have projected robust expansion for the generative AI market, with estimates suggesting substantial revenue growth in the coming years.
Ethical Implications of Generative AI
Generative AI has raised a number of ethical concerns. Some of the most pressing ethical implications of Generative AI include:
- Bias and fairness: Language models are only as good as the data they are trained on, and if the training data is biased, the resulting language model can perpetuate that bias. This can result in unfair or discriminatory outcomes for certain groups of people. Therefore, it is important to ensure that the data used to train language models is diverse and representative of all groups of people.
- Misinformation: Language models can generate realistic-looking text, which can make it difficult to distinguish between what is true and what is not. This can lead to the spread of misinformation or disinformation, which can have serious consequences, particularly in areas such as politics and public health.
- Privacy: Language models can generate text based on a user’s input, which can raise concerns about privacy. Users may be sharing sensitive or personal information with a language model without realizing it, and this information could be used for nefarious purposes.
- Autonomy and accountability: As language models become more sophisticated, there is a concern that they could be used to manipulate or deceive people. This raises questions about who is accountable for the actions of language models and how they can be held responsible for their behavior.
- Employment: The rise of Generative AI and language models could lead to the displacement of human workers in certain fields, particularly in areas such as customer service and content creation. This could have serious implications for employment and the economy.
Overall, there are many ethical considerations to be taken into account when developing and deploying Generative AI, and it is important to carefully consider these implications and develop policies and regulations to ensure that language models are used in a responsible and ethical manner.
Impact of Generative AI
Generative AI has the potential to revolutionize the way we interact with technology. With its ability to create new content, it has the potential to transform the industries listed above and more. However, with this potential comes the risk of misuse, and it is important to regulate and monitor the development and deployment of Generative AI.
Generative AI is an exciting new development in the field of artificial intelligence, and it has the potential to create significant positive impacts across various industries. As with any new technology, it is important to consider the risks and benefits and approach them with caution. The future of Generative AI is bright, and it will be fascinating to see how it continues to evolve and shape our world.
And One More Thing
In full disclosure, this article was adapted from a conversation with ChatGPT and as such was mostly generated by Generative AI.
Contact our team of experts to learn more about WEKA and how we can accelerate your generative AI development.