Meet Contextual AI
Changing How The World Works Through AI
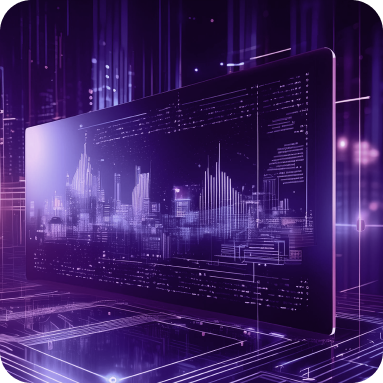
Headquartered in Mountain View, Contextual AI’s mission is to change how the world works through AI. The company provides a turnkey platform for building enterprise AI applications powered by its state-of-the-art RAG 2.0 technology.
Solving AI Model Hallucination and Currency
Contextual AI is helping to solve critical issues needed to enable enterprise AI at scale for Fortune 500 companies, including hallucinations, staleness, and data privacy. Large language models (LLM) are adept at generating clear and coherent responses based on their pretraining data but may lack critical context and timely information specific to a given use case to be impactful in production. When these off-the-shelf models lack proper context for a query or task, they confidently make false but plausible-sounding answers, known as hallucinations. Hallucinations compromise the accuracy and trustworthiness of the model’s answers and are thus a meaningful blocker to deploying AI into production in enterprises.
RAG 2.0: Driving Enterprise AI Adoption at Scale
Today, many developers leverage retrieval-augmented generation (RAG) to add external data to model responses in hopes of increasing the accuracy of their large language models. However, a typical RAG system today uses a frozen off-the-shelf model for embeddings, a vector database for retrieval, and a black-box language model for generation, stitched together through prompting or an orchestration framework. This leads to a “Frankenstein’s monster” of sorts. The solution is brittle, lacks domain-specific knowledge, requires extensive prompting and ongoing maintenance, and suffers from cascading errors. As a result, these Frankenstein RAG systems rarely pass the bar to make it into production.
Contextual AI’s CEO and cofounder Douwe Kiela led the team that pioneered Retrieval Augmented Generation (RAG) at Facebook AI Research (FAIR) in 2020. Today, he is developing RAG 2.0 with the team at Contextual AI to address the inherent challenges with the original RAG system.
The company’s approach focuses on two pillars: systems over models and specialization over AGI. Contextual Language Models (CLM) enable production-grade AI by optimizing the entire system end-to-end. With RAG 2.0, Contextual AI pre-trains, fine-tunes, and aligns all components as a single integrated system. As a result, customers can go from brittle generic chatbots to highly accurate and specialized AI applications, with improvements of over 4x compared to the baseline.
The Challenge
Generative AI workloads have demanding performance, efficient data management, and significant computational power requirements that can make them time and resource-intensive to train and serve. Contextual AI’s initial implementation was built in Google Cloud using the default storage offering, Google Filestore, but quickly ran into scale challenges and performance limitations, increasing costs and delaying its AI model development and training times. Contextual AI uncovered weaknesses in metadata handling, checkpointing, and data preprocessing, and data movement from storage to accelerator emerged as a key consideration for the team to drive faster AI model training times.
Long Data Load Times
The “lots of tiny files” problem creates challenges that most legacy storage architectures aren’t well equipped to handle. With LLM training, the model rapidly iterates to find the right file, open, read, close, and move on. A delay in load times of 10 to 20 seconds adds up to a substantial impact on developer productivity over a training epoch.
Long Model Checkpoint Write Times
Model checkpointing is essential to ensure resiliency during the training cycle, but it can cause AI models to stop training to complete the checkpoint. Really, really fast writes of a few very large model-weight files are key so that model training can continue. Long model checkpointing times meant the training would block for up to 5 minutes while the checkpoint was being written.
“Training large-scale AI models in the cloud requires a modern data management solution that can deliver high GPU utilization and accelerate the wall clock time for model development.”
The Solution
WEKA Data Platform on Google Cloud
Contextual AI relies on the WEKA Data Platform to manage all of its datasets for AI model training – totaling 100TB today. In this environment, WEKA software runs on a 10-node cluster of GCE C2-std-16 VMs, which provide a high-performance data layer built on the NVMe devices attached to each VM, amounting to 50 TB of flash capacity. The single WEKA namespace extends to an additional 50 TB of Google Object Storage, providing a scalable, affordable data lake to retain training data sets and the final production models.
“With the WEKA Data Platform, we now have the robust data pipelines needed to power next-gen GPUs and build state-of-the-art generative AI solutions at scale. It works like magic to turn fast, ephemeral storage into persistent, affordable data.”
Outcomes with WEKA
Contextual AI now has hundreds of model training epochs under its belt on the WEKA Data Platform. It has provided a big leap in data performance, leading to increased developer productivity and faster model training times.
The WEKA Data Platform combines low-cost object storage and high-performance flash-based storage in a single namespace and manages automated data tiering between them at a granular level. With a single copy of data, customers get the performance they need without over-provisioning storage resources. Contextual AI’s model checkpoint times have been made 4x faster, cloud storage costs have dropped by 38%, and developers are more productive.
3x
Performance Improvements
Contextual AI achieved a threefold increase in performance for key AI use cases thanks to a significant increase in GPU utilization.
4x
Faster AI Model Checkpointing
Eliminated delays in model checkpoint completion to achieve a 4x improvement in checkpointing processes, dramatically improving developer productivity.
38%
Cost Reduction
Associated cloud storage costs were reduced by 38 percent per terabyte.
Building Production-Ready Enterprise AI
Learn more about Contextual AI and WEKA in Google Cloud
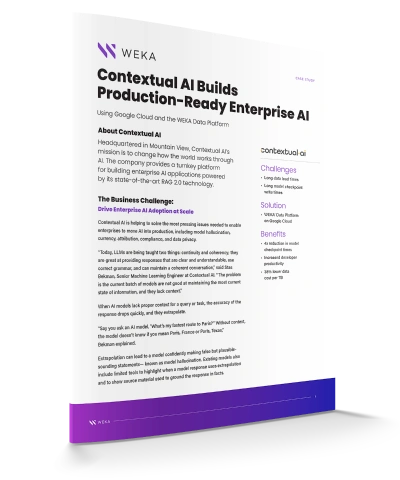