For the Want of a Nail – Part 1: How Infrastructure May be Limiting AI Adoption
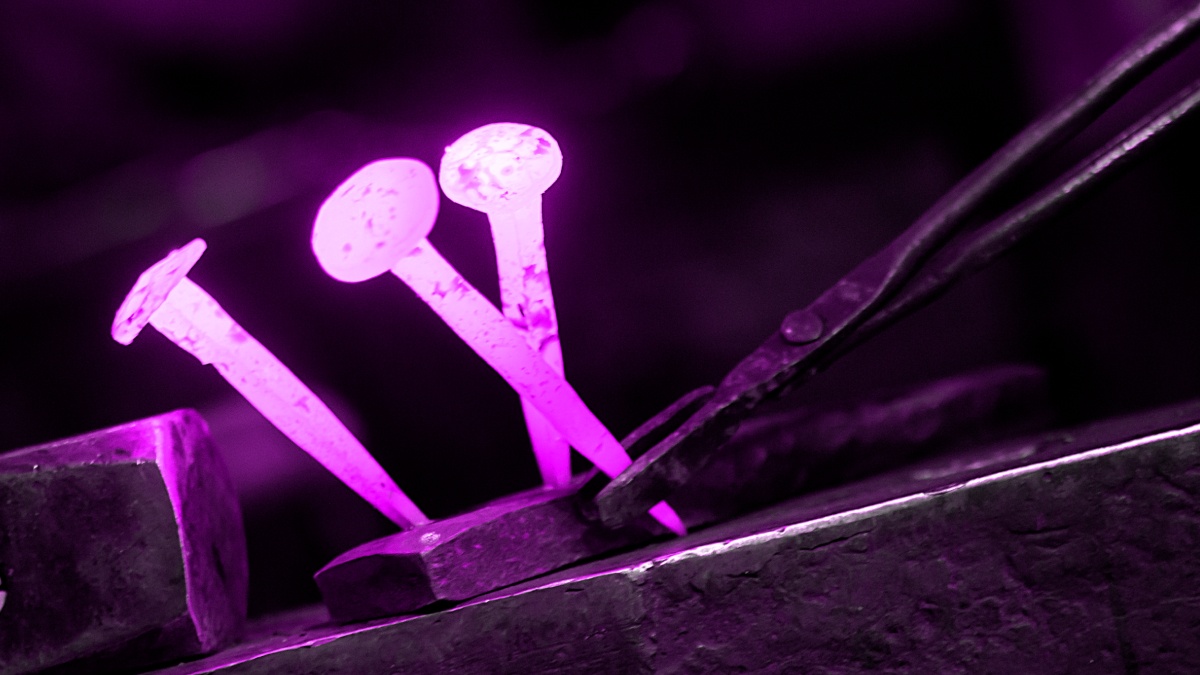
- For the Want of a Nail Part 2 – Aligning Data Center Storage with the Needs of AI Workloads
- For the Want of a Nail – Part 3 – AI Depends on Large Scale Storage
- For the Want of a Nail – Part 4 – Want AI? You’ll Need a Modular Approach to Maximize GPU Performance
- For the Want of a Nail – Part 5 – Enabling AI For Organizations Of All Sizes
Artificial Intelligence (AI) has come of age. It’s all around us. Yet many people don’t even recognize it and what it’s doing for us every day. There are many examples of cool stuff being powered by AI. Consider smart cars (Waymo, Uber, Mobileye); smart home devices (refrigerators, HVAC, Internet of Things (IoT)); virtual personal assistants (Siri, Cortana, Google Now); and Virtual Reality games (Call of Duty, Far Cry, etc.). There are also more practical AI applications such as expert systems (online customer support; purchase prediction, fraud detection, voice and speech recognition) as well as pattern and image recognition (identity confirmation/facial recognition, security surveillance, genome mapping, digital pathology, and so forth).
But perhaps most important, yet often out of the public view are the business applications for AI. The IoT is more than just remote-control refrigerators. It enables scenario simulation, fault prediction for business processes, monitoring and maintenance of industrial equipment such as early detection of gas leaks in pipelines. Frost & Sullivan estimates that AI clinical support will enhance medical imaging diagnosis to potentially improve patient outcomes by 30%+ while cutting treatment costs by up to 50%[1]. In 2014, an estimated 228 thousand human genomes were sequenced. By 2017 that figure is expected to jump to 1.6 million genomes, each representing hundreds of GB of data[2]. Machine learning can assist in preliminary drug discovery, clinical trial research, and next-generation sequencing[3]. Intelligent agents with machine learning enable companies such as Walmart to process high volume of transaction records within seconds[4]. Companies such as Pacific Specialty garnered new insights through a holistic view of data and analytics to anticipate customer needs and new underwriting opportunities.[5] Simply stated, AI can unleash the latent knowledge deep within an organization’s data stores to drive business success.
AI fundamentally changes business processes and re-envisions data usage, which in turn demands a far-reaching reconsideration of data management. Unlike traditional enterprise applications that are largely transactional by nature (they operate in a sequential and discrete fashion on small data sets), AI storage workloads are highly parallel with continuous-interrelated activities that act as feedback loops to one another. This is the crux of machine learning: continually iterating on data to gain new insight and knowledge. As a result, AI apps require tremendous amounts of compute and data, which challenges existing network, computer, and AI storage infrastructures. Although AI apps and their implementation will differ, they will have broad impact across all verticals/industries. Yet at present, few businesses have deployed AI at scale. A recent McKinsey report indicated that only 20 percent of surveyed organizations currently use any AI related technology at scale or in a core part of their businesses.[6]
With the potential of AI being so great, how can businesses overcome the infrastructure challenge? AI requires very high performance with low latency, in particular for AI storage. Traditionally, this has demanded expensive investments in legacy technologies; however, the volume of data and the performance required for AI is not viable on these architectures. This is part of the reason that the prevalence and scale of AI deployments have been limited. The good news is that technological advancement means that some needs have already been addressed. Compute challenges have been overcome through parallel workload processing, which is enabled by innovative technology such as NVIDIA GPUs. Likewise, network performance has been enhanced through solutions such as InfiniBand connectivity as delivered by Mellanox. This leaves Data Management and AI storage as two areas where innovative solutions are essential to ensure that AI initiatives reach their full potential.
By solving data management and AI storage challenges, AI initiatives could become within the economic reach of not only large enterprises but small and mid-sized organizations as well. While the breadth and depth of AI apps will vary by company size, the ability to deploy them economically at an appropriate scale would be a benefit for any organization. But how can data management and the underlying data center AI storage architecture be aligned with the needs of AI workloads? That’s the topic I’ll address in my next blog.
[1] “From $600 M to $6 Billion, Artificial Intelligence Systems Poised for Dramatic Market Expansion in Healthcare.” Frost.com. January 5, 2016. https://ww2.frost.com/news/press-releases/600-m-6-billion-artificial-intelligence-systems-poised-dramatic-market-expansion-healthcare.
[2] Hiatt, David. “The Next Digital Arms Race In Life Sciences.” The Next Digital Arms Race In Life Sciences. August 23, 2017. http://www.bio-itworld.com/2017/08/23/the-next-digital-arms-race-in-life-sciences.aspx.
[3] Mehta, Tapan. “Artificial Intelligence Euphoria in Healthcare.” Artificial Intelligence Euphoria in Healthcare. November 13, 2017. https://dminc.com/blog/artificial-intelligence-euphoria-healthcare-life-sciences/.
[4] Ruth, Joao-Pierre. 2017. “6 Examples of AI In Business Intelligence Applications”. Techemergence. https://www.techemergence.com/ai-in-business-intelligence-applications/.
[5] “Pacific Specialty Case Study”. 2016. Avanade.Com. https://www.avanade.com/~/media/asset/case-study/pacific-specialty-case-study.pdf.
[6] “Artificial Intelligence The Next Digital Frontier?”. 2017. Discussion Paper. McKinsey Global Institute. https://www.mckinsey.com/~/media/McKinsey/Industries/Advanced%20Electronics/Our%20Insights/How%20artificial%20intelligence%20can%20deliver%20real%20value%20to%20companies/MGI-Artificial-Intelligence-Discussion-paper.ashx.