How Do We Improve AI and Sustainability? Start by Rethinking the Modern Data Stack
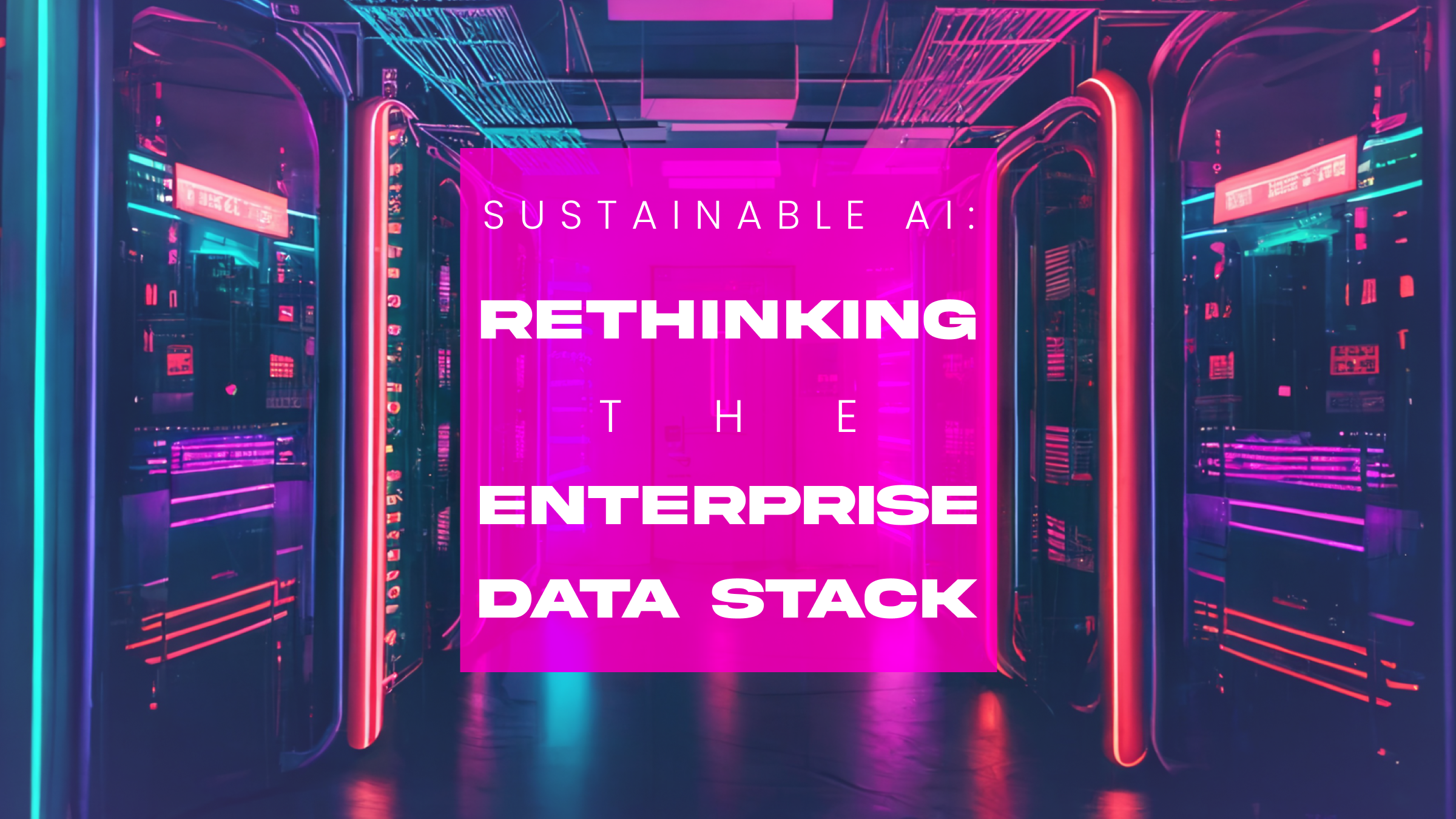
At WEKA, we believe adopting sustainable development practices is as important to running our business as building bleeding-edge innovation and a product that customers adore.
To wit, in February, we launched our Sustainable AI Initiative, focused on supporting industry discourse and problem-solving around what we’re calling the AI sustainability conundrum.
There’s no doubt that artificial intelligence (AI), machine learning (ML), and high-performance computing (HPC) have limitless potential to power good. In WEKA’s customer base alone, we’re seeing leading global enterprises and research organizations across a broad swath of sectors – including automotive, autonomous vehicles, manufacturing, media & entertainment, healthcare, pharmaceutical & drug discovery, space exploration, aerospace & defense, oil & gas, and government agencies around the world – making previously unimaginable gains in the pace of research, discovery, and breakthrough innovation. These intrepid organizations are harnessing the power of AI to help solve many of the most profound ecological, humanitarian and business challenges of our time while simultaneously transforming the way we connect, live, work, and play. And we’ve only just scratched the surface of what these technologies can help humankind to achieve.
As with any new transformative technologies, there is a fever pitch of conversation around AI’s potential and there is also a healthy dose of skepticism, suspicion, and concern. As I’ve noted before, the ethical considerations of AI and its applications are a well-worn topic in today’s media and political landscape. Rarely, if ever, though, do those conversations touch on how it is driving exponential increases in global power consumption and greenhouse emissions at a time when society must urgently find ways to reduce both.
Don’t get me wrong – AI’s potential for unintentional biases, invasion of privacy, or world domination by sentient digital overlords absolutely warrants further debate and governance. But its environmental impact must also become part of our industry’s responsible AI discourse. Even as we work to ensure generative AI doesn’t displace the human workforce or enslave humanity, it’s critical we are also considering how we can curtail its insatiable thirst for energy and growing carbon footprint.
Running to Stand Still
AI and ML models require a tremendous amount of energy to train and run. Today, 3% of global energy consumption is associated with the world’s data centers. That’s double what it was just 10 years ago. Without intervention, these next-generation workloads are expected to drive a threefold increase in energy demand by 2025.1
Translation: In the next two years, without sustainable AI practices, we can expect the world’s data centers to consume more energy annually than the entire human workforce combined.2
There’s no way society is going to put the AI genie back in the bottle – it’s simply too awesome and powerful and important – so we’re going to have to start working on AI and sustainability solutions for efficiency and productivity so we can minimize waste while extracting as much value as possible.
We believe the primary culprit perpetuating AI’s inefficiency is traditional data infrastructure and data management approaches, which aren’t equipped to support AI workloads or hybrid cloud architectures simply because they weren’t built for them. Just as you wouldn’t expect to drop the engine of a 1900s Ford Model T into the body of a modern Lamborghini or Maserati and have them perform as advertised, you can’t expect data infrastructure designed for last century’s data architectures to deliver the performance and latency requirements of next-generation technologies like AI and ML, which require a steady barrage of data moving at impossible speeds to run efficiently.
Legacy data storage is inherently tuned to address one data type or a specific performance profile, and as a result, the solution executes a copy strategy across multiple data points tuned for the right profiles, creating data siloes. This leads to management complexity, multi-copy redundancy, excessive storage and slower data access. All of which creates a significant processing overhead for AI workloads. It also leads to idling GPUs. Like a fossil-fuel guzzling motor vehicle idling at a stoplight, these digital transformation engines consume enormous amounts of energy and emit greenhouse gases for no reason. The answer is a zero-copy, zero-tune solution that allows you to store data only once, reducing wasteful idle and waiting time.
Rethinking the Modern Data Stack
In the era of cloud and AI, your enterprise data stack needs to be architected for hybrid cloud and software-defined. To harness next-generation workloads like AI, ML, and HPC, it needs to be capable of running seamlessly anywhere your data lives, is generated, or needs to go – whether that’s on-premises, in the cloud, at the edge, or in hybrid and multi cloud environments.
If that sounds familiar, it’s because it is. Yes, it plays neatly to WEKA’s value proposition – but not because it’s marketing lip service. Back in 2013, WEKA’s founders saw the impending storm coming for the data management industry with the advent of AI, ML, and HPC. They built the WEKA Data Platform as a cloud-native software solution to support these performance-intensive workloads efficiently and sustainably across edge, core and cloud.
The Rise of Data Oceans
Rethinking the data stack means it’s also time to rethink the data lake – a term used in the last decade or so to define a central location used to access data more efficiently without creating multiple copies of the same data for the purposes of extracting value.
Although data lakes have proven to be quite useful for unstructured data workloads not impacted by latency, GPU appetites for data often exceed what’s available in the average data lake and the typical protocol stack and what’s needed to fuel the large-scale data processing requirements of workloads like generative AI to achieve optimum efficiency.
Conceptually, it’s time to start architecting to support orders of magnitude larger datasets. Stagnant, siloed holding tanks of data need to become dynamic, frictionless waves of data that can be pipelined in a continuous steady stream to meet the insatiable data demands of AI more effectively and sustainably. This is not to imply that a data ocean is simply a larger holding tank for data; it also needs a dynamic levy and pipeline system to manage the “floodgates” of data servicing the ever-hungry cores of GPUs. Think of this architecture as a reservoir that supplies water to multiple constituencies of people through a network of controlled aqueducts, with water flowing continuously and without interruption to those who need it.
The Sustainable AI Forecast is… Partly Cloudy
Adopting at least some cloud in the modern data stack is a no-brainer. In a world where innovating with distributed teams and datasets increasingly being generated at the edge is rapidly becoming the new normal, public cloud environments provide customers with an efficient, flexible way to innovate at scale across multiple teams and locations.
It’s unrealistic to think the whole world can move everything to the cloud overnight, which is why we believe the future is hybrid. Although it would be nice to believe we could just flip a switch and make our on-premises data centers more sustainable, it’s not realistic. It’s time-consuming, expensive – and it’s a long game.
Migrating even some of your workloads to the cloud can make a big impact on your data operations’ carbon output and energy consumption in the short term, as cloud providers are building their hyperscale data centers to be ultra-efficient, and some are even fully powered by renewable energy sources.
We aren’t the only ones who think so: a recent study by McKinsey & Company cites: “With thoughtful migration to and optimized usage of the cloud, companies could reduce the carbon emissions from their data centers by more than 55 percent—about 40 megatons of CO2e worldwide, the equivalent of the total carbon emissions from Switzerland.”3
Now that’s a tangible impact.
There’s no silver bullet for AI and sustainability – at least not yet. It will require a multi-pronged approach and global action on many fronts. However, by collectively rethinking and modernizing how we manage data and optimizing it for next-generation workloads and applications, we believe organizations can begin to harness the power of AI more efficiently and sustainably.
Abatement of enterprise technology’s carbon and energy footprint is an area in which we all can – and must – act quickly to reduce global power consumption and greenhouse emissions. The horizon to course-correct is short and the clock is ticking. It’s imperative we all work with urgency to do our part to address it.
Learn More About WEKA’s Sustainable AI Initiative
1Association for Computing Machinery – Technology Policy Council: Computing and Climate Change | November 2021 https://dl.acm.org/doi/pdf/10.1145/3483410
2Gartner, Inc, October 18, 2022, Gartner Unveils Top Predictions for IT Organizations and Users in 2023 and Beyond https://www.gartner.com/en/newsroom/press-releases/2022-10-18-gartner-unveils-top-predictions-for-it-organizations-and-users-in-2023-and-beyond
3Source: McKinsey & Company,The green IT revolution: A blueprint for CIOs to combat climate change, Sept. 2022 https://www.mckinsey.com/capabilities/quantumblack/our-insights/the-state-of-ai-in-2022-and-a-half-decade-in-review