GTC 2025 Hot Takes: 10 Things You Missed If You Weren’t There
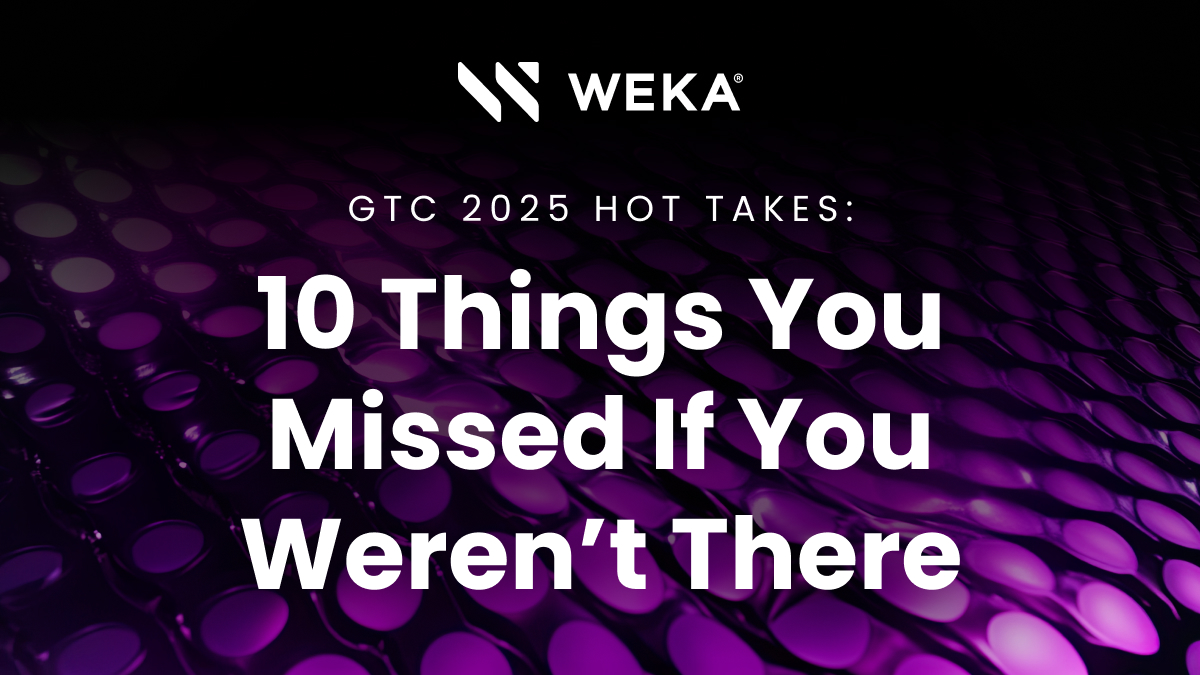
What happens in San Jose doesn’t have to stay in
San Jose.
NVIDIA’s GTC 2025 just wrapped—and if you weren’t there, don’t worry. We’ve got your back with the spiciest takeaways from the floor. From trillion-token AI futures to elevator-line innovation (yes, really), here’s what you missed:
Enterprise AI Is No Longer Coming of Age—It’s Here
Our primary takeaway from GTC25? Enterprise AI has officially gone mainstream.
Everywhere you turned, there were announcements of new partnerships and real-world deployments. This wasn’t just startups and academia anymore—this was:
- Global enterprises launching production-scale copilots
- Healthcare, finance, manufacturing, and telecom adopting AI-native pipelines
- Traditional software stacks integrating tightly with NVIDIA’s AI platforms
Inference at scale is now the true measure of enterprise AI success. This means deploying models, serving millions of requests per day, and managing cost, latency, and throughput in real-time. Companies are shifting from proof-of-concept to production and demanding infrastructure that can keep up.
Tokens Are the New Gigahertz
Today’s AI factories—massive, hyperscale data centers optimized for training and inference—already generate over a trillion tokens per second. And with agentic and robotic swarms expected to come online in 2025, that number is about to explode. We’re talking 86,400 trillion tokens per day.
Welcome to the era of token economics—where cost, efficiency, and speed per token become the key metrics for success. The smartest AI architectures won’t just be powerful—they’ll be token-efficient. That’s why the idea of a Token Warehouse™ is a game-changer. Don’t waste GPU cycles recalculating embeddings; store them, reuse them, recycle them, get lean.
Inference Is a Two-Part Dance: GPU and Memory
Speaking of, inference at scale is evolving. The first phase—disaggregated prefill—is GPU-bound. The second—decode—is memory-bound. You need flexible architectures that can independently and elastically provision GPU and memory resources, cloud-style. This disaggregation isn’t just smart—it’s critical to scalable, profitable AI.
Power as a Bottleneck Finally Being Talked About
AI is hitting power walls everywhere. GPU density and token throughput are scaling, but the power to support them? Not so much. We saw a new focus this year on efficiency per rack, efficiency per token, and minimizing data center waste. If your architecture can’t keep up with cooling and power delivery, all the GPUs in the world won’t save you.
This is where WEKA and others are stepping up—rethinking systems to maximize compute utilization while minimizing energy and space. Not just faster… smarter.
NVIDIA Is Now in the Systems Game—Big Time
GTC made one thing very clear: GPUs can’t power the AI revolution alone. With the GB200 Grace Blackwell, NVLink, NVSwitch, and focus on systems-level performance and introducing AI-Certified Systems Storage, NVIDIA is addressing the entire data pipeline. Memory bandwidth interconnects and data movement now stand shoulder-to-shoulder with raw GPU speed.
Bottom line: If your storage and networking can’t keep up, your AI can’t either.
Data Platforms Are Finally Getting Their Due
To build on that, NVIDIA openly recognizes that data platforms can significantly impact runtime performance.
It’s no longer just about model size or GPU speed—how data is staged, moved, cached, and reused is becoming a first-class consideration in AI infrastructure. Whether it’s training throughput or inference latency, data platforms are emerging as core levers for optimization.
For those building modern AI factories, data is not just input—it’s infrastructure. And platforms that can orchestrate it efficiently will define the next generation of AI performance.
Your Company Now Has an Evil Twin – Congrats
One of the most memorable moments from Jensen Huang’s keynote? He declared that every company will soon have two domains to manage: the physical and the digital twin.
This wasn’t just a future-facing prediction—it was a call to action. From factories and hospitals to cities and supply chains, digital twins are rapidly becoming essential infrastructure. Why? Because they enable simulation, automation, optimization, and AI-powered decision-making before deploying anything in the real world.
As the physical and digital continue to merge, managing your twin will be just as critical as managing your real-world assets. If you’re not building toward that future, you’re already behind.
The Expo Floor Was a Microcosm of the AI Revolution
The GTC expo hall this year was buzzing with energy (and, well, other things—see below). From foundational model vendors to robotics, edge AI to next-gen networking, it was inspiring to see AI permeating across every industry. It wasn’t just about chips—it was about how AI is being applied.
Pro tip: Don’t hit the expo hall right after lunch. The catered food might have been AI-optimized, but let’s just say the post-lunch atmosphere was a little… gassy.
More Inclusive Vibes Than Expected
One encouraging observation: noticeably better gender diversity than many other tech conferences. Data science (and maybe AI more broadly?) appears to be less of a tech bro monoculture. More of this, please.
Trade Show Innovation: Solving Elevator Line Hell
The wait for meeting rooms was next level. Elevator lines were longer than the keynote line. The result? Creative customer meetings in hallways, staircases, coffee lines—you name it.
We might need AI to optimize spatial matchmaking and vertical transit scheduling. NVIDIA, are you listening?
Final Take
If GTC 2025 had a theme, it was this: AI isn’t just about raw horsepower anymore. It’s about systems, scale, sustainability, and intelligence. Tokens are the new compute currency, power is your rate-limiting step, and system architecture is your competitive edge.
See you in the Token Economy.