How to Manage Data in the AI Era – Accelerated DataOps
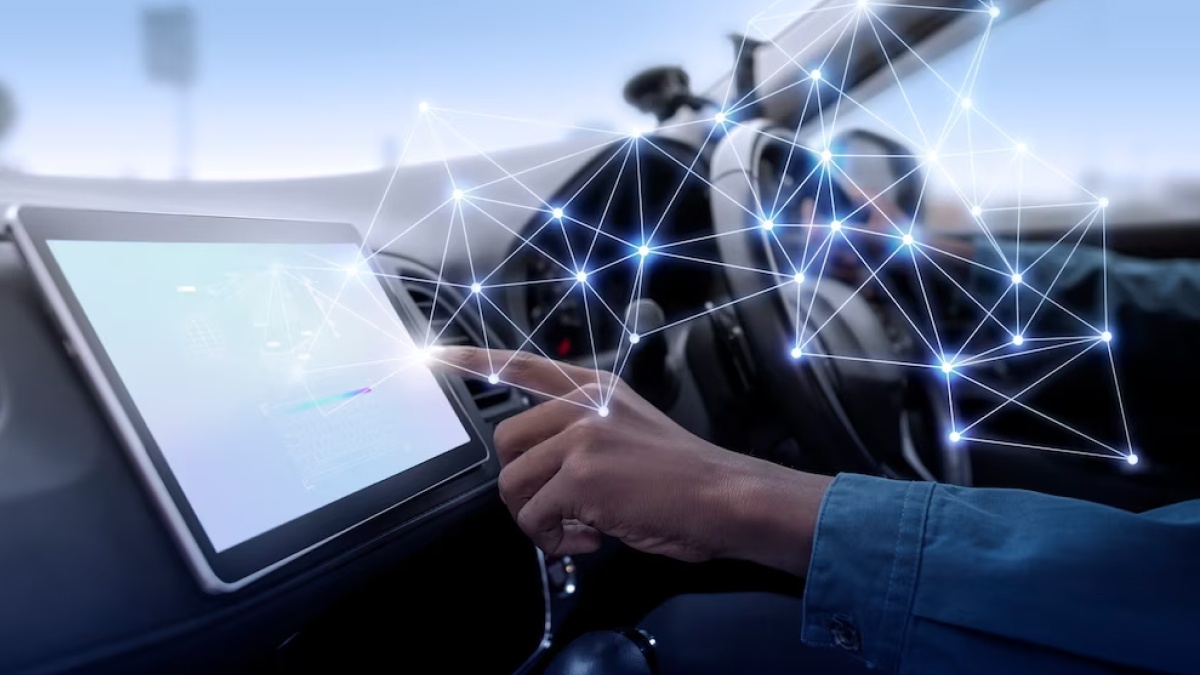
- The Convergence of Business and IT – Accelerated DataOps, Part 2
- Accelerated DataOps Paves the Pathway to Explainable AI, Part 3
Shailesh Manjrekar, Head of AI and Strategic Alliances at WekaIO, shares his perspective on Accelerated DataOps with Weka AI in Part 1 of a three-part series titled “Accelerated DataOps with Weka AI for Edge to Core to Cloud Pipelines.”
Introduction
Weka is excited to be launching Weka AI, a transformative solution framework for Accelerated DataOps. In my three-part series leading to our launch of Weka AI, I will explain the rationale as it relates to new workloads, new architecture, and finally Weka AI and the customer benefits it provides.
Accelerated DataOps – It’s all about the data!
Data has become the most important strategic asset to digital businesses for launching new business models, faster time to market, and competitive differentiation. Accelerated DataOps – Data Management in the AI era – determines how well businesses can derive actionable intelligence, operationalize pipelines, and provide governance and trust. It is going to determine success in the digital economy.
New Workloads – Convergence of HPC, HPDA, and AI with Accelerated Computing
AI is penetrating the traditional High-Performance Computing (HPC) and High-Performance Data Analytics markets. For example, HPC customers are leveraging GPU-accelerated INDEX libraries for simulation on GPU compute, with WekaFS-enabled GPUDirect Storage. RAPIDS and BlazingSQL can run GPU-Accelerated Data Analytics and GPU-Accelerated SQL querying, and with the DALI library, run Deep Learning on GPUs. The Accelerated Compute layer is not just limited to GPUs but involves FPGAs, Graph processors, and specialized accelerators.
The use cases are moving from computer vision to Conversational AI, NLP / NLU, and multi-modal use cases. Recommendation engines from Alibaba and Baidu are now using deep learning, while you see low latency inference used for personalization (LinkedIn), speech (iFLyTek), translation (Google), and video (YouTube) use cases.
Learning (Training) is quickly moving from supervised (labeled data) to using Convolution Neural Networks (CNNs) for annotation and labeling, to transfer learning (where one Deep Neural Network (DNN), trained on one set of datasets, can also be trained for other datasets), to federated learning (centralized), to active learning (label selective scenarios). Similarly, DNNs are becoming more and more complex, as with the several billion hyper-parameters of BERT and Megatron.
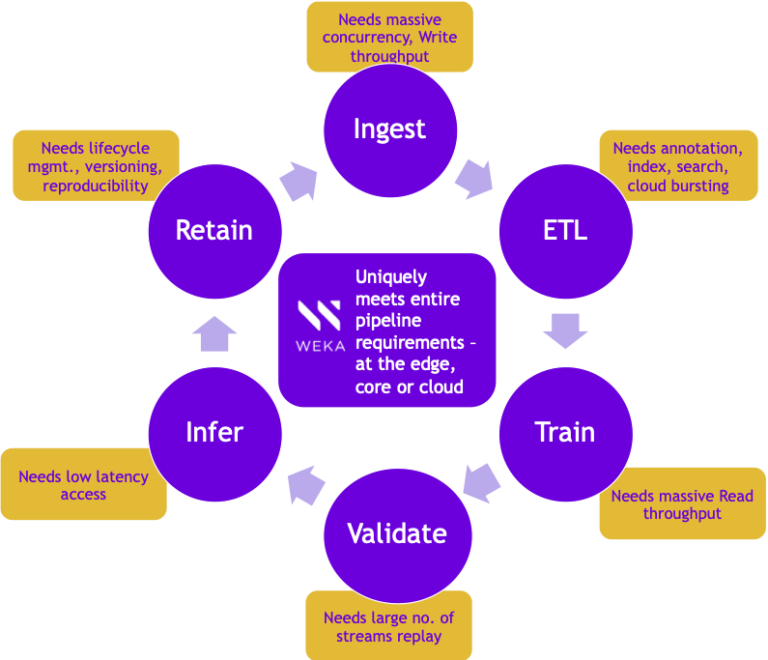
All of these transitions result in different stages within AI data pipelines that have distinct data (storage and I/O) requirements for massive ingest bandwidth, mixed read/write handling, and ultra-low latency, often resulting in a storage silo for each stage. This means that business and IT leaders must reconsider how they architect their storage stacks and make purchasing decisions for enabling Accelerated DataOps.
Summary
Weka, an NVIDIA Partner Network Solution Advisor, is uniquely positioned to anticipate the needs from these market transitions and provide transformative solutions. Weka, through delivering these solutions, makes it easy for our customers to monetize their data, achieve faster time-to-market, and gain competitive differentiation with the best TCO. Stay tuned for Part 2 of the series “Accelerated DataOps with Weka AI for Edge to Core to Cloud Pipelines,” where I outline the transitions underlying datacenter architectures, and Part 3 on how Weka AI is able to cater to these market transitions.
To learn more about WekaIO’s solutions for Artificial Intelligence and Data Analytics, click here.