For the Want of a Nail – Part 2: Aligning Data Center AI Storage with The Needs of AI Workloads
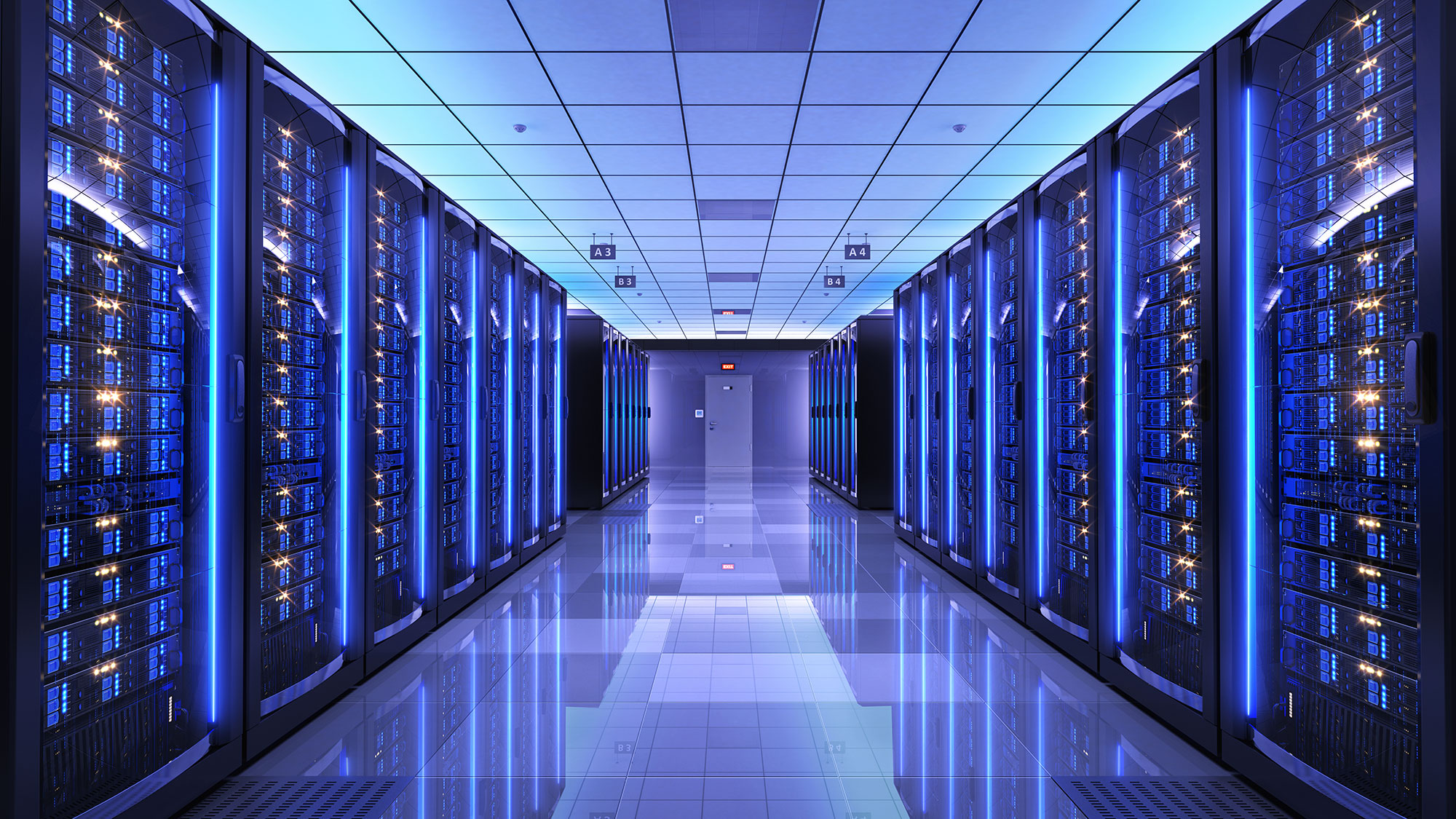
- For the Want of a Nail Part 1 – How Infrastructure May Be Limiting AI Adoption
- For the Want of a Nail – Part 3 – AI Depends on Large Scale Storage
- For the Want of a Nail – Part 4 – Want AI? You’ll Need a Modular Approach to Maximize GPU Performance
- For the Want of a Nail – Part 5 – Enabling AI For Organizations Of All Sizes
As I discussed in my previous blog, Artificial Intelligence (AI) fundamentally changes business processes and provides potentially life altering results by unleashing the latent knowledge deep within an organization’s data stores. Of course, discovering latent knowledge requires an aggressive approach to find, unlock, and leverage its associated business value. In other words, there is a lot of work associated with AI — it’s more than just having the right applications — it’s about having the right infrastructure that can cost-effectively support them.
Deploying AI in data centers challenges existing network, computer, and AI storage infrastructures with workloads that are parallel in nature with interrelated activities that continuously act as feedback loops to one another. According to Topbots[1], there are twelve essential categories of AI vendors: business intelligence, productivity, customer management, HR & talent, B2B sales & marketing, consumer marketing, finance & operations, digital commerce, data science, engineering, and industrials & manufacturing. As you might imagine, unlocking knowledge (and business advantage) requires sifting through lots of data, and perhaps across formerly siloed disciplines. If your storage and data center administrators are already pushed to the limit maintaining the status quo, just imagine their reaction to the needs of AI.
The key design consideration for AI storage infrastructure is the ability to deliver high bandwidth with low latency for both small and large files. Consider an AI workload that supports the monitoring and maintenance of industrial equipment. This entails periodically reading log files, which themselves are usually small in size. Yet the aggregated log data for all the equipment on the shop floor, if not multiple sites, could yield a huge data set. Conversely, an image/pattern recognition workload might be examining hours of surveillance data searching for the moment when the unauthorized removal of an item from the premises occurred.
Another example involves self-driving cars, which require real-time processing of image and censor data to help navigate common driving hazards such as roadway construction, traffic, emergency vehicles, and poor driving conditions. While much of this data and its processing will occur in-vehicle, a great deal of data will also need to be transferred and stored for use later by manufacturers to improve decision making algorithms and by insurance companies for liability purposes. Consider for a moment the volume of data that would be generated by a fleet of delivery vehicles or rental cars; it’s easy to see the impact on such as enterprise.
This raises an issue beyond file size and AI storage capacity. In traditional environments, compute and data are typically housed in a centralized data center. Therefore, the distance and hence latency of interconnections can be quite low. However, the Internet of Things (IoT) is predicated upon data that can be distributed far away from compute resources. For real-time applications, low latency is essential for smooth operations. The highly distributed and parallel nature of AI applications demand high bandwidth at low latency, regardless of file size and location.
Traditional parallel file systems such as IBM Spectrum Scale, Lustre, Hadoop, and others support highly coordinated concurrent access from multiple clients while featuring optimized I/O paths for maximum bandwidth. As a result, these files systems can increase throughput and scalability for large files. However, they are not designed with smaller files and many metadata tasks, and generally do not offer the same performance associated with large files. In addition, these file systems are 20 years old and do not take advantage of the benefits offered by advanced technologies such as GPU processors and NVMe flash memory. At the same time, parallel file systems are extremely complex because there are many moving parts (metadata servers, multiple storage targets, tunable system parameters, etc.) that require ongoing optimization to run at peak efficiency. Data management in such environments is a nuanced and ongoing specialized task that is typically beyond the capability of a traditional storage administrator. As a result, such installations require dedicated, skilled architects and administrators, which can be a non-trivial expense.
As you are probably starting to realize, a successful AI storage deployment will require a rethinking of existing infrastructure in order to maximize your AI efficiency and ROI. But this is just the start. Artificial Intelligence is driven by large amounts of data, yet the result of AI is even more data. The ability to scale AI storage with the growth of AI data is essential for success. In my next blog I’ll discuss how success depends upon large scale AI storage. I invite you to join me there.
[1] Jia, Marlene. ” The Essential Landscape of Enterprise AI Companies”. March 31, 2017. Accessed November 07, 2017. https://www.topbots.com/essential-landscape-overview-enterprise-artificial-intelligence/
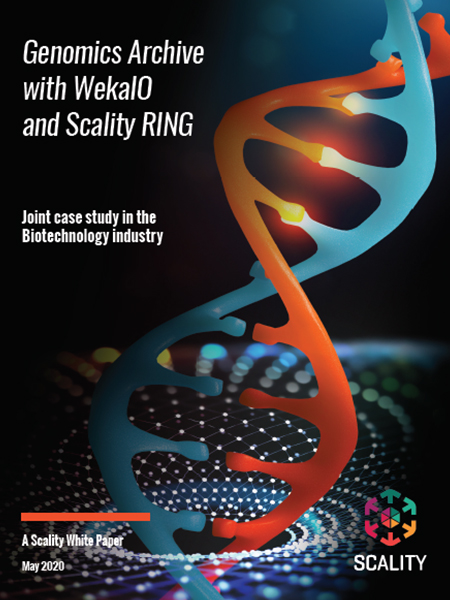
AI AND HIGH VELOCITY ANALYTICS NEED A NEW FILE SYSTEM
Lectus arcu bibendum at varius vel pharetra vel. In cursus turpis massa tincidunt.