Top 7 Things You Need to Know About Data for Generative AI
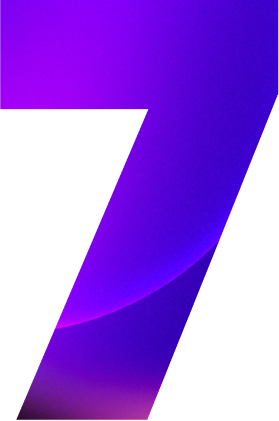
Things You Need
to Know About Data for Generative AI
Generative AI has made remarkable strides in generating realistic and creative content such as images, music, and text. The key to unlocking the full potential of generative AI models lies in efficiently using high-quality, diverse training data. However, this cutting-edge technology also poses several data-related challenges.
Here are the top 7 things you need
to know about data for generative AI models.
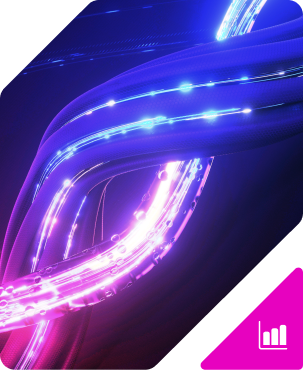
1. Quality and Quantity
Generative AI models heavily rely
on large amounts of high-quality data.
The more diverse and representative the data is, the better the model’s ability to generate meaningful outputs, but there are trade-offs in terms of time and cost. Larger data sets take longer to train and require more expensive infrastructure,
so finding the sweet spot between the two is key.
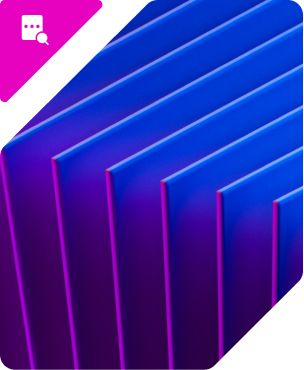
2. Data Preprocessing
Preprocessing plays a crucial role in preparing the data for generative AI models.
Tasks like cleaning, normalizing, and transforming the data are essential to remove noise, standardize formats, and make the data suitable for training the model.
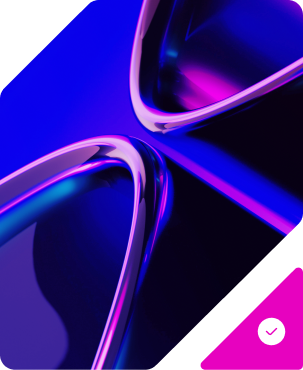
3. Training Set vs. Validation Set
Splitting your dataset into training and validation sets is vital.
The training set is used to train the model, while the validation set helps assess the model’s performance and tune hyperparameters. Optimize for each step separately and look for solutions that help them optimize without a ton of overhead.
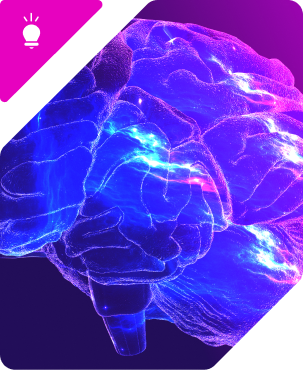
4. Augmentation Technique
Data augmentation techniques can enhance the generative AI model’s performance by artificially increasing the dataset’s size and diversity.
Techniques like rotation, translation, scaling, and adding noise can help generalize the model’s learning and improve its ability to generate novel outputs.
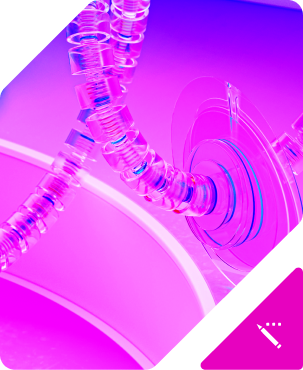
5. Labeling and Annotation
Depending on the generative AI task, labeling or annotation of the data may be necessary.
For example, in image generation, each image may need annotations for objects, attributes, or classes. Properly labeled data enables supervised learning and helps the model understand patterns and generate relevant outputs.
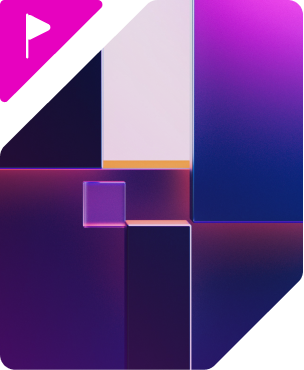
6. Bias and Fairness
Generative AI models can inadvertently learn biases present in the training data, leading to biased outputs.
It’s important to address bias and ensure fairness by thoroughly examining the dataset, identifying potential biases, and implementing mitigation strategies during preprocessing and model training.
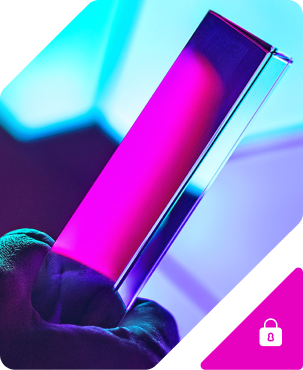
7. Data Privacy and Security
Generative AI models may require sensitive data, such as personal information or proprietary content.
It’s crucial to handle data privacy and security carefully. Implement appropriate measures like data anonymization, access controls,
data governance, and encryption to protect sensitive information and comply with relevant regulations.
Remember,
staying updated with the latest research and best practices in data management will help you make the most of these powerful models.