AI Storage (The AI Storage Solutions You Need For Complex Data)
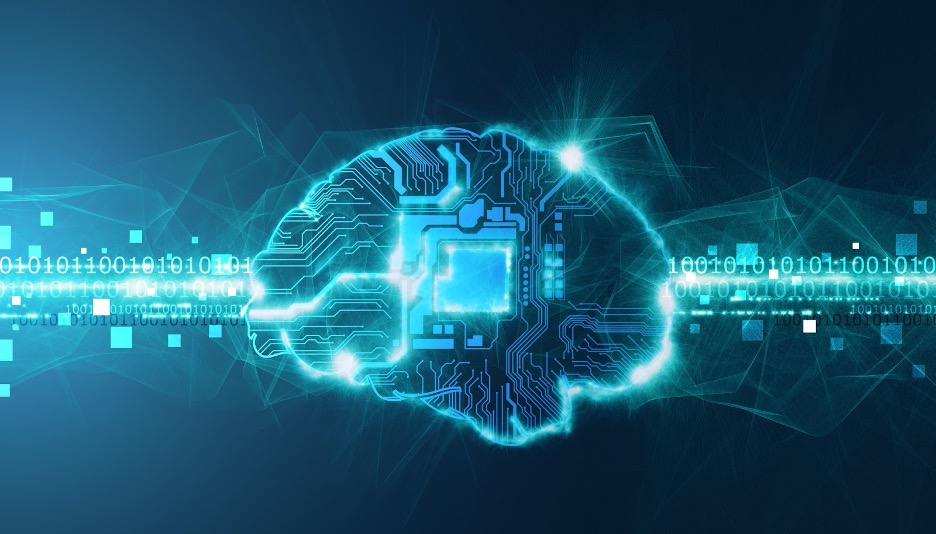
Were you wondering about AI storage? We discuss what is AI storage, the challenges caused by data-intensive applications, and the AI Storage solutions you need.
What is AI Storage?
Artificial intelligence requires massive amounts of data that the cloud environment must process in real time and store for later use. This requires an AI data storage solution that is high performance, flexible, and scalable.
How Does AI Storage Work?
Artificial intelligence and machine learning applications rely on data to function. Large volumes of data power training simulations and models inform decision-making and increase the effectiveness and accuracy of any insights.
Not only must AI applications have large volumes of data on hand to function, but also that data must be accessible through rapid, reliable, and scalable storage solutions. Hence the invention of AI storage, or data storage tailored explicitly to machine learning and AI workloads.
Such storage for AI must include a few critical features to function as a component of AI workloads effectively:
- Rapid Access from Data Center to Cloud Edge: AI is often built on complex cloud infrastructure. Storage, applications, and data collection apparatuses may be spread across a cloud environment. AI storage will support rapid access to data across the entirety of the cloud, regardless of where it is or where it is going.
- Automation: Storage earmarked for AI workloads will necessarily have several key functions associated with it, including dynamic management of file and resource permissions; identity and access management configurations; routing and load balancing plans and processing directives. AI storage requirements will automate these functions to streamline their operations in the system better. Appropriately, this automation may also be powered by AI.
- Scalability and Performance: AI storage must scale. Period. While workload demands may fluctuate over time, the storage for AI workloads must quickly respond to these demands without hindering those workloads. Storage can quickly become the bottleneck in such applications, and proper storage can mitigate slowdown or scaling issues.
- Cost and Efficiency: AI applications are large in scope and scale, and mismanagement of resources can lead to increasingly problematic costs. AI storage solutions should provide some level of cost-effectiveness, either through bulk storage management or proper scalability, to avoid purchasing and maintaining resources that aren’t used.
While this list isn’t exclusive, the combination of effective storage management, cost management, and scalability are the foundation for AI storage.
What Are Some Components of an AI Storage Architecture?
AI storage and cloud environments will call for certain system configurations, particularly when it comes to optimizing hardware and data transfer infrastructure. Storage will function as part of a high-performance computing environment composed of several crucial components.
These components of AI Storage Architecture will include the following:
- GPU-Accelerated Hardware: The rapid processing of data from storage into machine learning and AI algorithms calls for hardware that can handle massive, parallel computing tasks. Cloud infrastructure with built-in GPU acceleration can support such tasks, which will optimize the storage, transmission, and processing of the volume of data needed to support AI applications.
- High-Performance Computing Clusters: HPC clusters combine shared storage, file systems, and servers that can rapidly scale and support heavy workloads associated with AI and machine learning. A properly configured HPC cluster that draws from fast local storage to prepare data for use in massive AI algorithms is central to building more advanced artificial intelligence.
- NVMe Flash Storage: With such large workloads, it’s important to have fast storage retrieval available at the hardware level. NVMe storage, close to the GPU, can serve as an immediate storage medium for AI apps that need incredibly fast data access.
- Solid-State Hard Drives: AI storage can also include longer term storage outside of NVMe flash. However, systems are increasingly moving away from spinning disk media for any storage application outside of archives or long-term storage. More systems are turning to solid-state storage to provide another layer of data access that can cut down access times and improve overall performance.
When it comes to AI storage, the most important factor is speed. Fast flash and solid-state storage, combined with optimized hardware, facilitate that need.
What Are the Advantages of AI Storage?
Properly configured, AI storage provides several benefits beyond just powering AI applications. Such storage shapes the effectiveness of those applications and the effectiveness of connected systems and services.
Some of the advantages of optimized AI storage include the following:
- Advanced Analytics and Data Insights: AI applications often drive business insights and system analytics for massive cloud and IT infrastructure. Optimized AI storage can help support those analytics, but more importantly, optimized HPC and hardware can make AI apps more accurate, insightful, and responsive to real-time data changes.
- Accurate Reporting and Alerts: Additionally, accurate real-time data availability can support how AI algorithms report and alert system administrators about problems in the system. The availability of such data as well as the computing resources to process it means faster and more accurate reporting across a system.
- Resiliency, Failure Prediction, and Recovery: Optimized storage will typically operate using automation to support key management features. Such automation can also help AI storage infrastructure to better respond to problems. This includes documenting and responding to issues with data transmissions, disk integrity checks, routing failovers within HPC clusters, and automating backups.
- Agility and Flexibility: AI storage, powered by and powering artificial intelligence, is going to provide a level of agility that human-administered systems cannot match. AI can optimize storage for use in incredibly high-demand workloads while using that storage to feed machine learning. These systems can more readily and rapidly respond to real-time issues, demands for scaling, and system adjustments due to technical issues.
Power AI Applications with WEKA and AI Storage
Applications using or training artificial intelligence cannot rely on traditional storage to function properly. These applications need high-performance, high-availability storage from which they can continuously draw high volumes of data to fuel learning and growth.
WEKA supports high-performance cloud computing for machine learning and AI workloads, including high-performance AI storage using optimized hardware and cloud configurations.
Some of the features included with WEKA include:
- Streamlined and fast cloud file systems to combine multiple sources into a single high-performance computing system
- Industry-best, GPUDirect Performance (113 Gbps for a single DGX-2 and 162 Gbps for a single DGX A100)
- In-flight and at-rest encryption for governance, risk, and compliance requirements
- Agile access and management for edge, core, and cloud development
- Scalability up to exabytes of storage across billions of files
If you’re ready to get started with your own cloud-enabled AI projects and need a dedicated and tailored high-performance cloud system to support them, then contact WEKA and learn how we can help.
Additional Helpful Resources
How to Rethink Storage for AI
Six Mistakes to Avoid While Managing a Storage Solution
IDC Report – Requirements of a Modern Storage Platform
Data Management in the Age of AI
Genomics and Cryo-EM Workflows in Life Sciences
Architectural Considerations for AI Workloads
What is Data Orchestration? A Guide to Handling Modern Data